Statistics for Data Science: How to Learn Just Enough in Weeks
A few weeks of learning can help you stand out from the crowd in the long run
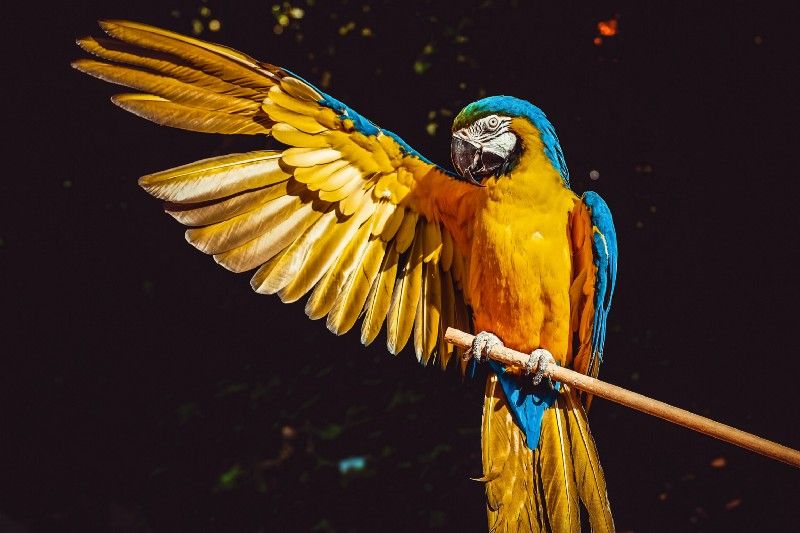
“What is your weakness?” the interviewer fired the age-old typical question.
“Statistics for data science”, I replied without thinking twice.
I can see their eyebrows raised with concern. They were not expecting this. “So what did you do about it?” they inquired, exactly the way I planned this to go.
I wasn’t going to go down that path unless I had a fitting response, duh.
“As you know, I come from a computer science background. I’m good with maths, programming and machine learning. That was enough for me to break into data science.” I paused.
“I knew some basic statistics, but I hadn’t heard of the Central Limit Theorem, so that doesn’t really count. For the last few months, my focus has been on improving my knowledge of statistics by following online courses, and I must say I’ve improved tremendously. You can now ask me about the Central Limit Theorem.”, I responded with a laugh.
I did end up getting the job offer, and that was how I turned a weakness into a strength. I didn’t lie — I really was weak in statistics. I found that out by myself before someone else pointed it out, which made all the difference.
If you’re reading this, chances are you’re not yet confident with your statistics for data science. You might have even skipped it to focus on machine learning and programming as you started. I was like you too, but it doesn’t have to be like this.
Read on to know how you can learn just enough to turn your weakness into a strength in a few weeks.
Statistics with Python For Your Rescue
After I realized my weakness, I went into a secret learning mode. I wanted to learn statistics that are relevant to data science and from a reputed university.
After reviewing multiple courses and getting opinions from colleagues with statistics backgrounds, I settled on the excellent Statistics with Python Specialization offered by the University of Michigan.
It took me about 6 weeks of after office hours to complete this 3-course specialization, but hey, it was worth all the time and effort I patiently invested in this. I wished I got about it much earlier because I slowly started understanding all the statistical concepts.
The course is packed with many examples, case studies, and exercises that are helpful for a beginner. The lectures started with the basics and gradually increased the complexity. They covered:
- Understanding and Visualization Data: All the stats basics you’ll ever need like study design, data management, visualizing data, interpreting different types of data and data sampling.
- Inferential Statistics: Lessons focused on learning principles behind using data for estimation and for assessing theories.
- Fitting Statistical Models to Data: The final course is focused on the science and art of fitting statistical models to data, including making inferences about relationships between variables and generating predictions for future observations.
I felt this specialization was more than sufficient to understand statistics commonly used in the industry, and you must gain clarity on these topics in your early days.
You Can’t Master Statistics Until You Do This
The certificate you get after completing the courses isn’t of any use unless you can confidently challenge the interviewer to ask any question.
The confidence only comes when you have applied statistics in real-world scenarios. Designing and analyzing a survey, evaluating hypotheses and making statistical claims and so on.
Understanding the theory behind this is one thing, but applying them in real-world scenarios is another.
There were plenty of projects and assignments given as a supplement to the course — give it a go without skipping them. It will be a great starting point if you don’t have access to real-world statistics intensive projects at work.
For my masters, I created a survey and collected responses. I analyzed the responses, formed hypotheses, validated them with statistical significance and made concluding remarks. The truth is, one doesn’t need to be enrolled in masters to do it.
You can’t master statistics for data science unless you apply what you learned.
This is How You Learn Just Enough Statistics for Data Science
You skip statistics because it doesn’t seem so important. You learn all the maths, programming and machine learning. You build projects and create a portfolio. You even apply for jobs and finally break into data science.
Then you realize your weakness: you know nothing about statistics. You feel like an imposter. You secretly start working on it before someone else notices it.
You pick a reliable online course from a leading university and learn it after work. You apply what you learn through projects and solidify your concepts. You revisit Central Limit Theorem multiple times, just in case.
When you feel confident, you go and expose your weakness only to turn it into a strength.
This, my friend, is how you learn just enough statistics in a few weeks.
As a note of disclosure, some affiliate links have been used in this article to share the best resources I’ve used and at no extra cost to you.
For more helpful insights on breaking into data science, honest experiences, and learnings, consider joining my private list of email friends.